Benefits and pitfalls of using machine learning to mitigate customer churn
Product leader James Gunaca relates his experiences of using machine learning to address customer churn.
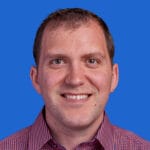
James Gunaca
Product Career Coach | Founder @ Product Sphere | Ex-Amazon | Award-winning Manager | Author & Speaker @ MindtheProduct at Product Sphere
Read more by James »
Comments
Join the community
Sign up for free to share your thoughts