Imagine a team that recently released a new feature—users are adopting it, growth is on target, and initial feedback is positive. However, after a few weeks, they begin to notice a drop in growth and retention. Despite users liking the feature initially, something seems to be off, but the team doesn’t have the right metrics to understand what’s happening. They realize they have relied on simple adoption numbers and vague satisfaction surveys, which don’t provide enough insight into why engagement is declining or how to address it. This story is all too common when teams over-rely on the powerful yet surface-level metrics such as usage and satisfaction. Moving toward more evidence-based decision-making requires better metrics, this is how.
Modern product management teams, often trained in MBA programs and coming from business backgrounds, are taught John Doerr's philosophy that 'what gets measured, matters.' While this is fundamentally true, it also creates a risk: choosing the wrong metrics can lead teams gravely astray. If a team focuses on metrics that are easy to track but lack relevance to user or business outcomes, they might miss the underlying reasons for success or failure, leading to misguided decisions.
It is no wonder that usage and satisfaction are common default metrics for teams. They are the most straightforward to calculate and come as defaults within most product analytics tools. Yet, while they are valuable at sharing whether someone chose to engage with a feature or flow and if they enjoyed the experience, they do little to suggest whether a person found that solution effective at what they were trying to accomplish. When we remember that products are tools intended to satisfy the needs of our users, it becomes more clear that we should actually measure whether they do exactly that.
At this point, you are likely thinking, "Well, if it was that easy, I would already be doing it." If this is you, the missing link is likely a strong definition for your feature. When each additional component added to a product has the outcome that it is intending to create for a user and how it will specifically change their behavior to achieve that, the team inherently has new success metrics possible. Creating these definitions can be completed using many approaches stemming from Jobs to be Done and Design Thinking. My approach is called the User Outcome Connection and is a simple three-part framework. Through this lens, a well-defined feature has specific behaviors that are being changed, which leads to a positive impact on the user and ultimately drives successful business outcomes.
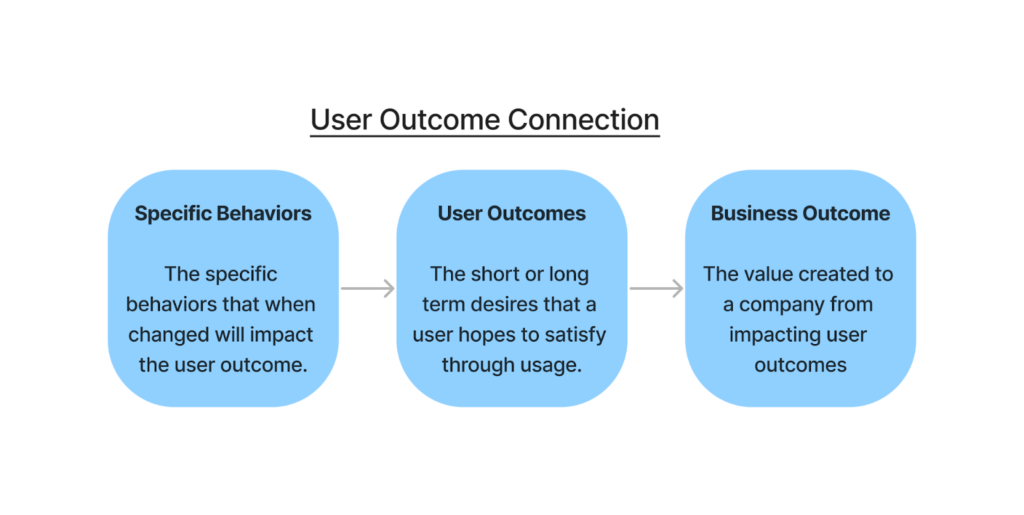
With a well-defined feature using the User Outcome Connection framework, teams can establish a clear understanding of the intended user impact. This clarity allows them to expand their success metrics and create variables that accurately reflect the feature's effectiveness. The Success Metric Framework builds on this foundation, helping teams go beyond surface-level data to capture meaningful impact. Here are its core components:
To illustrate this, examples are shown for the Grammarly Performance Score and the Ninjago Security Training Features. Both showcase how metrics at each level can be created.
Grammarly's performance score evaluates the accuracy of a document by analyzing writing issues and comparing it to similar documents, providing users with insights to improve their writing quality.
HeyMarvin's research repository is a centralized platform that streamlines the collection, organization, and analysis of qualitative research data, enhancing collaboration and efficiency for research teams.
With the five levels of success metrics in mind it is time to go and begin the process yourself. The following four steps are a guide to get you from selected features through the actual creation of the metrics. In these steps you will see the desired approach is creating ideal metrics first and then scoping them down to reality.
Identify core features: The first step in effective metric creation is identifying which features are the most impactful. Determine what each feature is trying to achieve and define the expected outcomes clearly. Understanding the purpose of a feature will guide the process of choosing meaningful metrics that can reflect its success or areas for improvement.
Build out definitions: Once the core features are identified, build out detailed definitions by creating complete user outcome connections for each feature. This involves specifying what behaviors are expected to change, how those changes will benefit the user, and how they ultimately support business goals. It is essential to ground these definitions in research wherever possible, as it ensures that the proposed outcomes are realistic and that there is evidence to back up your assumptions.
Scope out ideal metrics: After defining the user outcome connections, the next step is to develop metrics for each component of the connection. Start by thinking about what ideal success would look like—what metrics would best represent that success without any technical constraints? This creates a 'north star' vision, helping teams understand what is possible. Developing functional metrics can be challenging, but utilizing domain expertise alongside Generative AI tools can significantly assist in identifying potential indicators that align with the intended outcomes.
Build realistic proxies: Ideal metrics are not always feasible to implement due to technical, resource, or data constraints. When ideal metrics are out of reach, build realistic proxies. Proxies should still align closely with the desired outcomes but may be simplified or indirect measurements that are easier to implement. Remember, it's often better to have a good proxy metric than to measure nothing at all. The key is to ensure that proxies provide enough insight to make informed decisions about feature success and necessary adjustments.
Defining features clearly through the User Outcome Connection framework and creating metrics across the five levels of success metrics is essential for driving impactful decisions. By focusing on usage, usability, behavioral changes, user outcomes, and business outcomes, teams can ensure they are measuring what truly matters. Instead of defaulting to what's easy, defining meaningful metrics will provide the insights needed to guide product success, improve user experiences, and achieve strategic business goals.
Comments
Join the community
Sign up for free to share your thoughts