In the fast-paced world of SaaS product management, it's easy to get caught up in the excitement of innovation. We chase the next big feature, the groundbreaking solution, all while navigating the ever-shifting landscape of user expectations and market trends. But sometimes, the most significant lessons come not from moments of triumph, but from quiet reckonings with the hidden complexities beneath the surface.
Such was the case for me when the ethical and legal implications of historical data use truly hit home. It wasn't a eureka moment of technical brilliance, but rather a sobering conversation with our legal team that brought the issue into sharp focus. We had a wealth of historical data collected from user transactions, data that had been explicitly consented to for those specific purposes. But the question arose: did that consent extend beyond those initial transactions to other unforeseen uses we might envision down the line?
This seemingly simple question exposed a crucial oversight: the tendency to view data as a freely available resource, ready to be repurposed in our pursuit of product improvement. It forced us to confront the ethical and legal gray areas surrounding historical data use, and the potential impact on user trust and privacy. More importantly, it served as a stark reminder of the importance of building products with ethical considerations woven into the very fabric, not bolted on as an afterthought.
This seemingly simple issue isn’t so obvious; it's the kind of situation that can hit any of us. Imagine you are working on a new feature for your “Fitness App” product. The goal: leverage all that existing fitness tracking data to start recommending products to users. It sounds brilliant – increased user engagement, a potential new revenue stream – a total win, right? Well, not so fast. Here's where things get complex:
By sharing my own experience, I hope to spark a conversation among fellow product managers about how to navigate such uncertainties during the “cool AI/ML features” you are thinking about. Let's move beyond the “free data” mentality and explore ways to be responsible stewards of user information. Through collaborative dialogue and shared learnings, we can ensure that our AI/ML integrations in SaaS are not just technologically innovative, but also ethically sound and legally compliant.
With these insights in mind, one of the first and most critical areas we'll explore is the legal and regulatory landscape surrounding AI in SaaS.
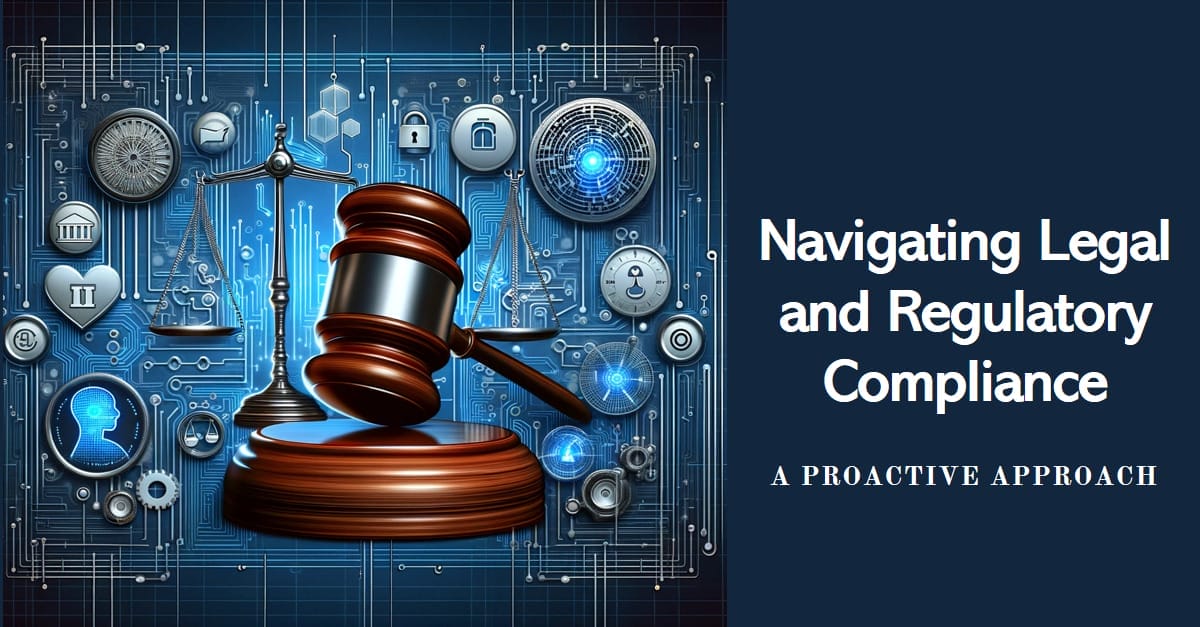
Integrating AI in SaaS is not just a technological endeavor but also a legal and ethical one. Our experience has highlighted the critical need for close collaboration with legal teams, particularly in the compliance review stage of AI projects. A pertinent example is IBM's decision in 2020 to withdraw its facial recognition software, citing concerns over potential misuse and racial profiling [1]. This move reflects a deep understanding of the societal implications of AI and the importance of adhering to ethical standards.
To navigate the complex legal landscape, product managers should:
By taking these proactive steps, product managers can ensure their AI solutions are not just innovative and effective but also legally sound and ethically responsible. It’s about being ahead of the curve, anticipating potential legal challenges, and embedding a culture of ethical awareness within the AI development process.
Having established the importance of legal and ethical compliance, let's delve into another foundational aspect of ethical AI: Data privacy and security.
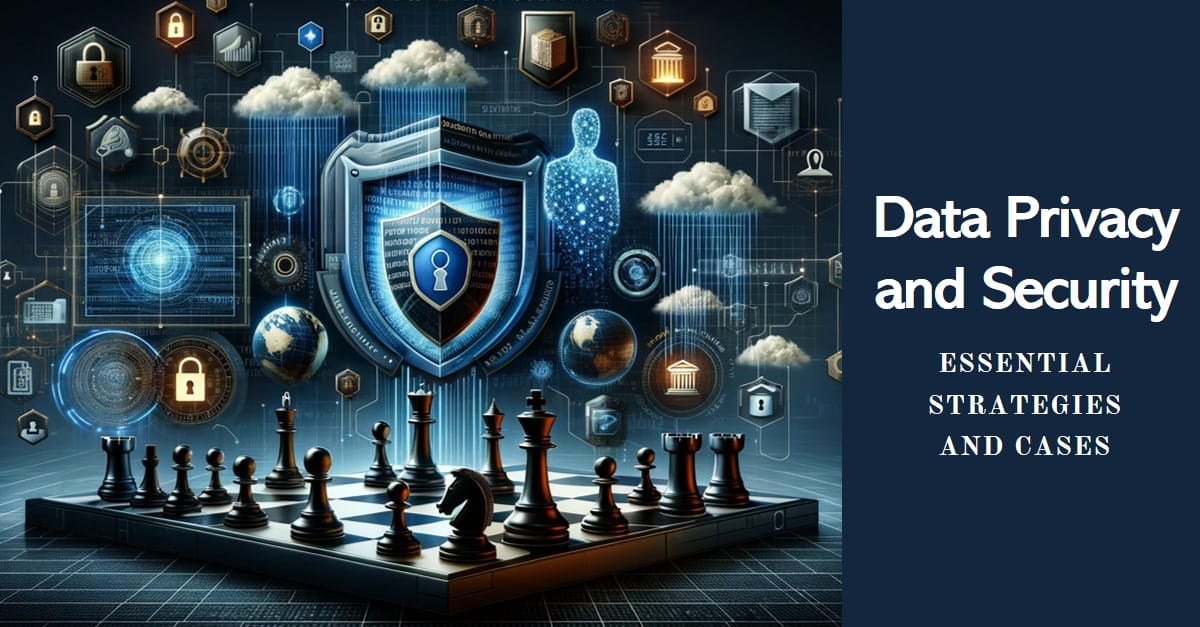
In the domain of AI-driven SaaS products, safeguarding data privacy and ensuring robust security are paramount. The case of Marriott International's GDPR violation in 2020, resulting in a hefty £18.4 million fine [2], stands as a potent lesson. It underscores a crucial point: mere compliance isn't sufficient; a proactive stance on data security is vital.
For product managers, crafting a secure and privacy-respecting AI environment involves several critical steps:
In summary, the lesson from the field is clear: robust data governance, a proactive privacy stance, and the utilization of advanced security measures are not just best practices; they are essential in the ethical deployment of AI in SaaS solutions. By implementing these strategies, product managers can ensure their products not only comply with legal standards but also earn the trust and confidence of their users.
While ensuring privacy and security is crucial, another significant challenge in ethical AI is addressing bias and fairness, which we will explore next.
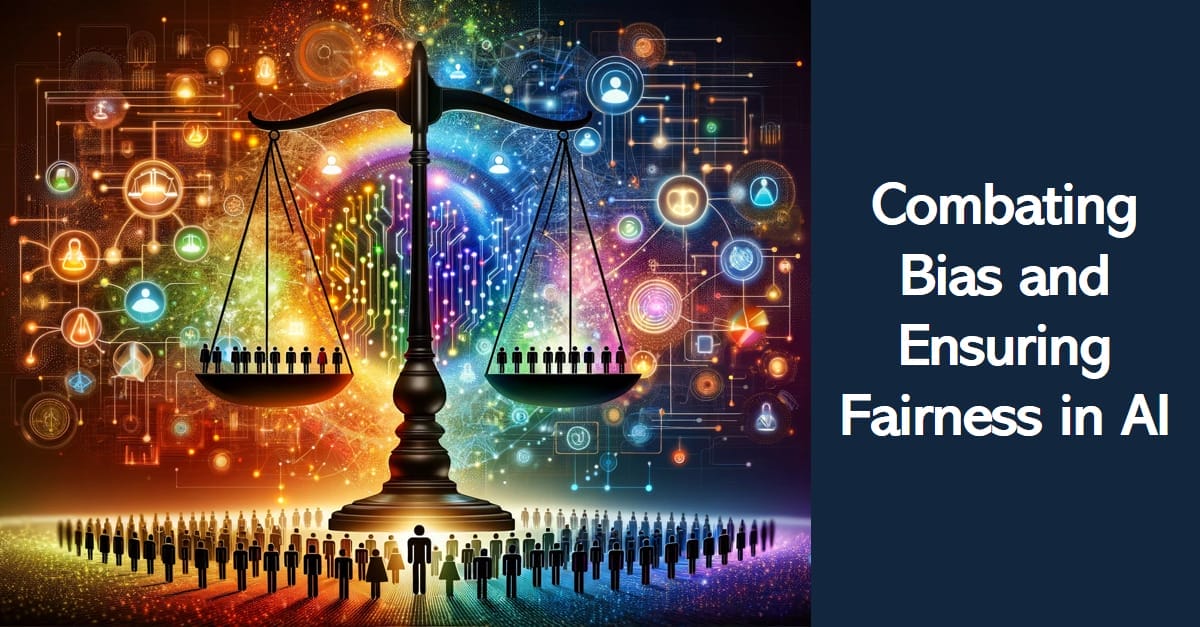
The challenge of bias in AI is a critical issue that every product manager in the SaaS sector must confront. Our understanding deepened when we encountered unintended bias in our own AI models, a scenario not uncommon in the field. A notable instance was Microsoft's AI chatbot, Tay, in 2016, which rapidly assimilated and reproduced biased and offensive language from user interactions [3]. This incident serves as a stark reminder of the potential repercussions of unchecked AI systems.
To combat bias, it's essential to adopt a comprehensive strategy that involves continuous monitoring and updating of AI systems. This includes:
Additionally, tools like Google's What-If Tool offer valuable insights into how algorithms impact different user groups. Such tools can be instrumental in identifying unintended consequences and ensuring that AI systems treat all users fairly. Incorporating these practices ensures that AI systems in SaaS products are not only technically proficient but also equitable and just, fostering trust and reliability among users.
Beyond internal system dynamics, it's equally important to consider the broader societal impact of our AI solutions.
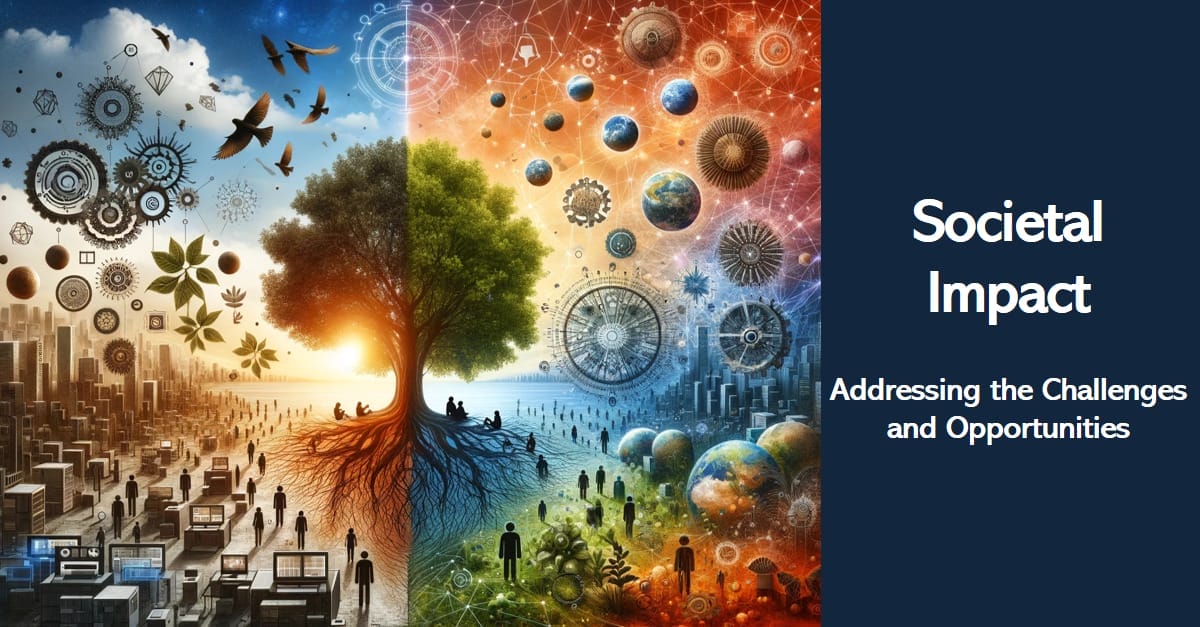
The implementation of AI in SaaS extends beyond technical feats, touching upon the broader societal fabric. A profound realization of our responsibility emerged when we considered the potential job displacement due to automation. Amazon's response to this challenge, through its Upskilling 2025 program, is an exemplary case. By committing over $700 million to train 100,000 of their employees in new skills, Amazon has set a standard for how companies can address workforce transitions in an AI-driven future [4].
This approach demonstrates that companies can and should play a pivotal role in mitigating the negative impacts of technological advancement. Beyond job displacement, there are opportunities for AI to contribute positively to society. Google's AI for Social Good initiative is a testament to this [5]. The program leverages AI to address significant global issues such as environmental conservation and education, showcasing the potential for AI to be a force for good.
For product managers, this means:
The societal impact of AI is multifaceted, and as product managers, it's our duty to navigate these complexities, ensuring that our innovations not only advance technological frontiers but also positively shape the society we live in.
As we've navigated through the various facets of ethical AI in SaaS, a recurring theme emerges: the profound responsibility that we, as product managers, shoulder in this innovative yet challenging domain. Our decisions today will not only shape the AI technologies of tomorrow but also redefine our interactions and ourselves.
Our aim should not be mere compliance or the pursuit of quick successes at the expense of ethical considerations. Instead, we should apply our knowledge and empathy to build AI solutions that are not only technically groundbreaking but also ethically sound and socially responsible. This journey demands courage, collaboration, and an unwavering commitment to doing good. Together, we can ensure that AI becomes a force for positive change, empowering individuals, enriching communities, and propelling humanity towards a brighter future.
Comments
Join the community
Sign up for free to share your thoughts