Integrating Generative AI into existing workflows can feel like an intimidating leap, especially for organisations unfamiliar with its potential. Our recent project took place within a large global advertising company. With a vast array of products and teams operating independently across the organisation, there’s a constant challenge of connecting these silos.
Our work focused on enhancing the core omni-channel media planning platform- a global centralised tool, supported by many regional instances. Our goal was to test GenAI’s capability by embedding it into the planning tool to enhance the “Brief to Segment” process. Specifically, we aimed to improve campaign efficiency and targeting relevance by generating audience recommendations from descriptive campaign briefs, ultimately saving planners time and boosting effectiveness. Here’s a look at our journey, from proof of concept to scaling, and some of the lessons we learned along the way
The project kicked off with a three-week proof of concept aimed at augmenting an existing advertising planning tool. The objective was to build a feature that could quickly translate campaign briefs into targeted audience segments, saving time for planners and boosting campaign effectiveness. The team was particularly interested in leveraging GenAI not just as a technological showcase but as a means to deliver real value to end users as swiftly as possible.
However, we quickly encountered challenges. The available audience segment descriptions were limited and often lacked the depth needed for effective targeting. To address this, we developed a data enrichment pipeline, enhancing the descriptions and providing more comprehensive inputs for the LLM to reference when generating recommendations. This step proved pivotal in improving the relevance of the outputs, setting the stage for scaling
Our GenAI solution leverages Retrieval-Augmented Generation (RAG) to enhance media planning. This approach provides more accurate and contextually rich suggestions compared to basic LLM-based methods.
Retrieval-Augmented Generation (RAG) is a method that combines the strengths of information retrieval and generation models to enhance the capabilities of language models. Here’s a breakdown:
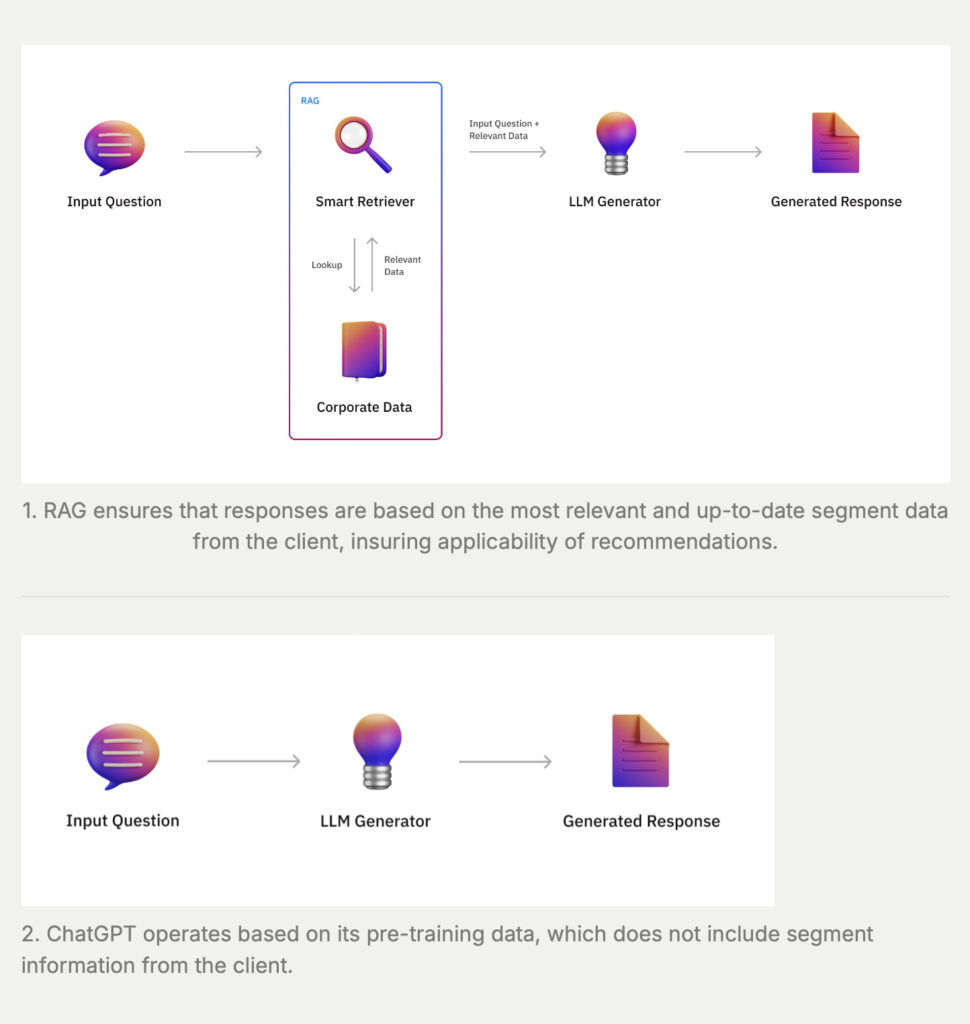
Product management in GenAI isn’t a straight path—it’s navigating constant shifts and roadblocks. During this project, our proof of concept quickly evolved into a more complex integration with the existing tool. But progress wasn’t linear. We faced unexpected data restrictions that forced us to switch data providers mid-project, and continually adjusted to stakeholder demands and market shifts. Staying flexible wasn’t optional; it was essential. We leaned into ambiguity, adapting rapidly without losing sight of our goals, showing that embracing uncertainty is part of building innovative products in an evolving landscape.
We emphasised early delivery and began backend development while finalising designs. We created a high-level plan with estimated timelines to ensure feasibility and visualised the delivery approach. At the start of each sprint, we set flexible goals, allowing engineers to research and implement solutions without rigid planning. We prioritised documentation after development to avoid wasted effort. Our small team facilitated quick discussions and alignment, minimising the need for formal knowledge transfer.
The limitations of the initial data set were an early blocker. The available audience segments were fairly narrow in description, often failing to provide the context the LLM needed for accurate recommendations. To address this, we built a data enrichment pipeline that expanded and detailed these segment descriptions, giving the model more relevant content to work with. This step was more than a technical improvement; it was a strategic shift that allowed us to retain control over the outcomes. By providing admins with oversight before the outputs reached end users, we ensured alignment with our goals and maintained quality standards."
This became even more critical when we were informed that the segments we had trained on were no longer accessible to UK planners due to legal restrictions. We had to quickly pivot to a new data provider, underscoring the importance of having flexible processes that can adapt to dynamic environments.
Evaluating GenAI products in B2B settings requires metrics beyond standard click-through rates. We focused on output quality, contextual understanding, and user satisfaction, but establishing a baseline was challenging due to the client’s recent platform migration. To address this, we implemented a feedback mechanism and captured user briefs, though engagement in the PoC was lower than expected. In the future, LLM as a judge capability could serve as quality evaluators, helping us set benchmarks. Additionally, exploring measures of employee productivity or satisfaction could provide further insights. As GenAI projects scale, articulating the value delivered is essential.
Navigating internal dynamics is often one of the biggest hurdles for any product manager, and our experience was no different. With established AI teams and innovation centres within the organisation, there was a clear need to position this GenAI solution as an enhancement to existing workflows rather than a competing initiative. We emphasised that our goal was to augment the current processes and empower planners with better tools, rather than replace existing methodologies. This approach helped in securing stakeholder buy-in and aligning the project with broader organisational goals.
There were significant concerns about the accuracy of our AI-generated audience segment recommendations. This was particularly pronounced among the Data Science team, who worried about the potential for errors.
To address concerns about output quality, we implemented a multi-faceted approach. First, we incorporated human control by allowing marketers to review and select audience segments generated by the LLM. This ensured that inappropriate recommendations were filtered out. Second, we used an LLM as a judge to assess the quality of the generated descriptions, providing a confidence score for each. This helped administrators prioritise segments for review. Finally, we acknowledged that while our solution couldn't guarantee 100% accuracy in every case, the benefits outweigh the risks, especially considering the time constraints faced by our users.
Proving the concept quickly through a targeted, small-scale pilot allowed us to iterate based on real user feedback without overcommitting resources. This approach kept the project agile and focused on delivering immediate, measurable value.
Positioning the GenAI capabilities as a way to enhance current processes rather than as a new tool, helped in securing stakeholder buy-in and minimised resistance. By integrating AI thoughtfully, we were able to add value without disrupting established workflows.
Navigating unclear and changing requirements is an inherent part of product management in GenAI. Our project’s success depended on staying adaptable, managing evolving needs, and being prepared to pivot when necessary.
The integration of GenAI into media planning isn’t just about adopting the latest technology; it’s about delivering value where it matters most. By starting small, enhancing existing workflows, and remaining flexible amidst uncertainty, we were able to build a solution that improved campaign effectiveness and set the foundation for future scaling. As product managers, our role is to guide these innovations thoughtfully, ensuring they serve both the immediate needs of users and the broader goals of the organisation.
As we look to the future, the landscape of Generative AI will continue to evolve rapidly. By embracing new technologies like synthetic users and exploring untapped use cases, we can continue to push the boundaries of what’s possible, making AI an integral part of driving business success and innovation in the years to come.
Comments
Join the community
Sign up for free to share your thoughts