,
Artificial Intelligence (AI) & Machine Learning (ML)
APR 24, 2019
Talking About Data Science by Jenessa Lancaster
Recommended
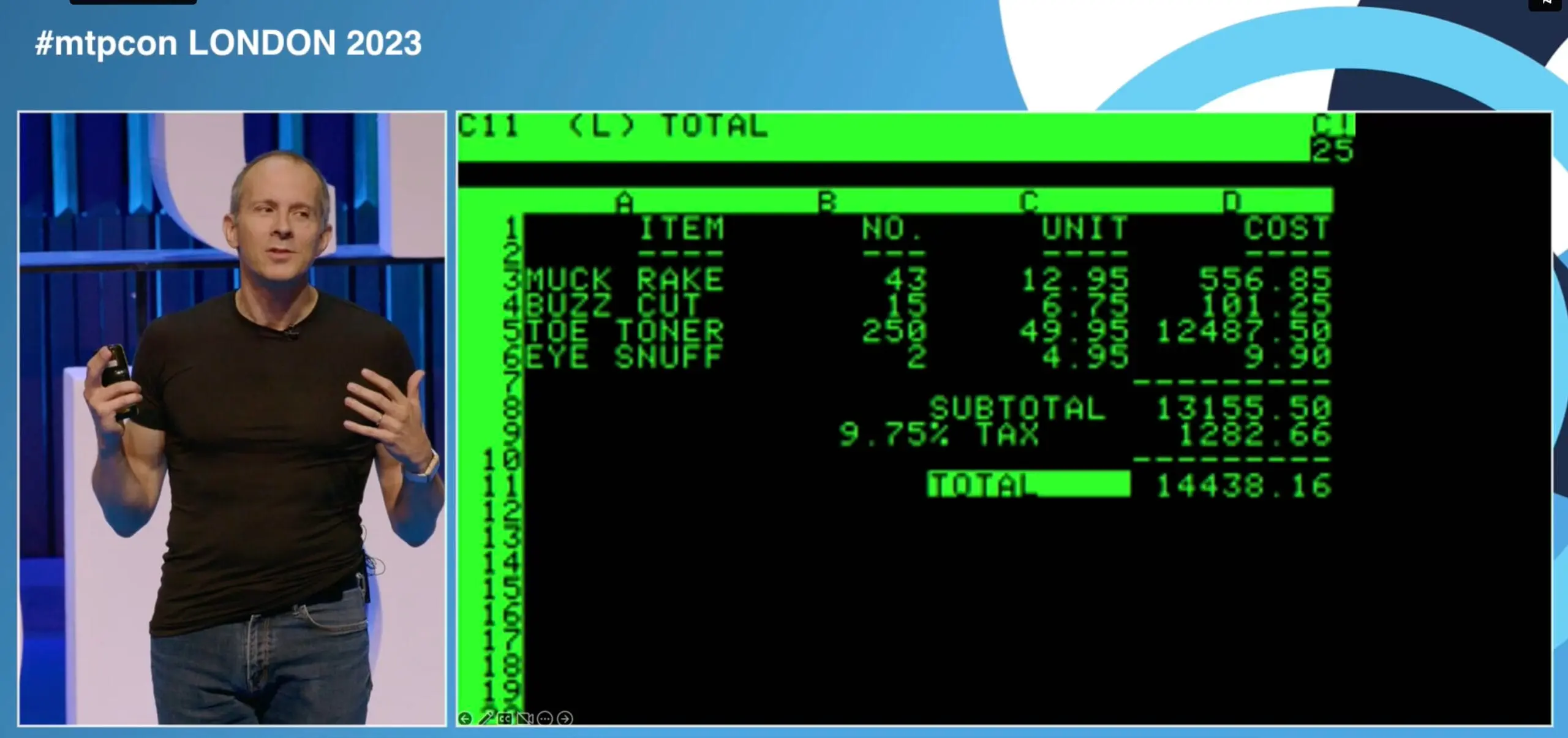
43:50
What we get wrong about technology by Tim Harford

24:28
How to keep your head about generative AI (when everyone is losing theirs) by Claire Woodcock